AUGMENTED INTELLIGENCE
Across every industry, organizations are investing in artificial intelligence (AI) and machine learning (ML) to unlock business insights from their data. Unfortunately, many leaders struggle to scale their AI/ML models into production across the enterprise.
Worse still, organizations spend precious time and resources monitoring and retraining models. Teams often can't replicate successful ML experiments, and data scientists don't have access to the technical infrastructure they need to innovate.
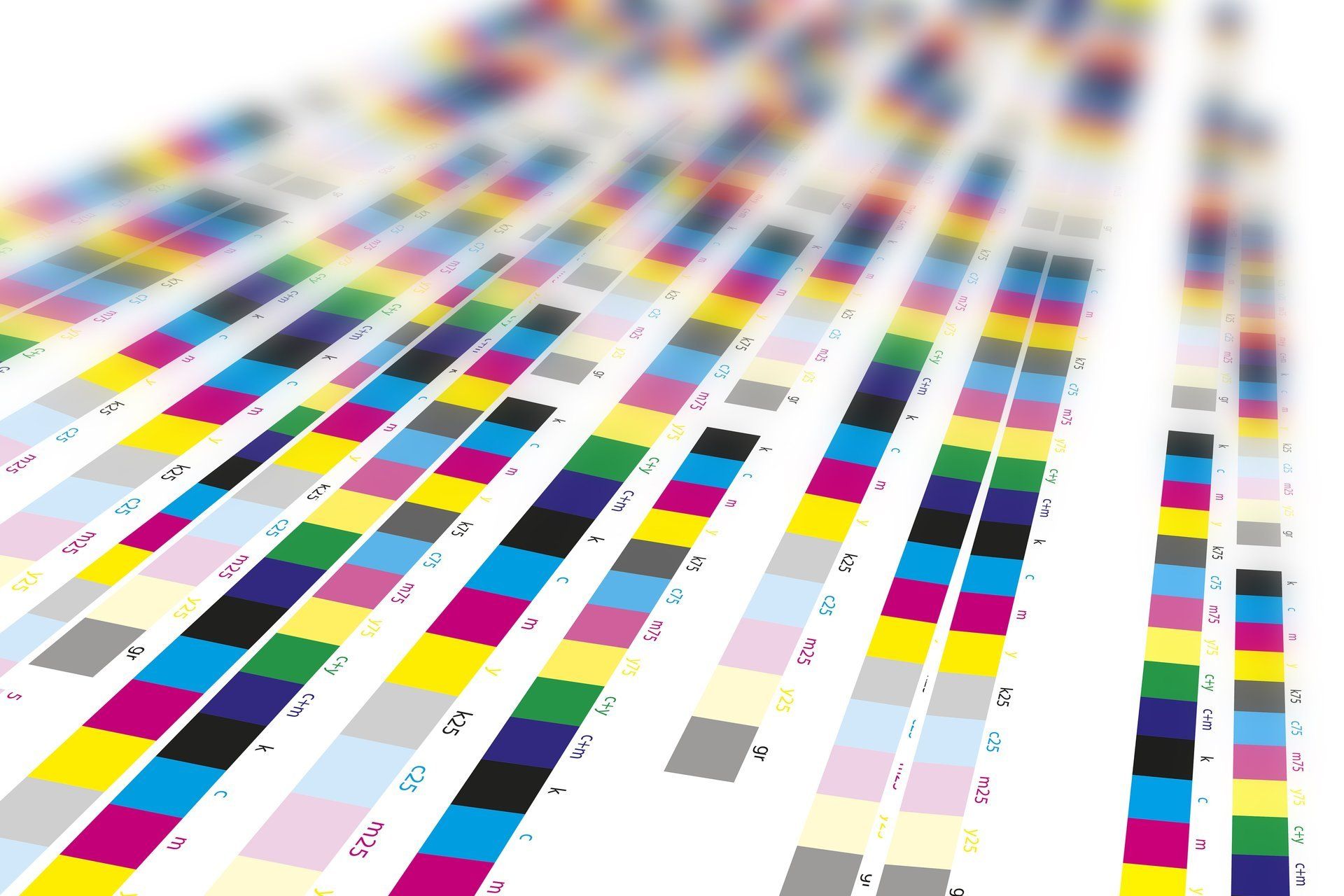
Build your plan
When you understand all the areas of MLOps maturity, you can develop a questionnaire to evaluate your organization. All questions must carry a weighting that, when collated, will help you establish if your organization is of low, medium, or high maturity – in other words, a laggard, early adopter, or leader. Here's how:
- To assess people maturity, question how well you include the necessary data science and business leaders across all stages of the ML project lifecycle
- For process maturity, determine how you've organized your data fabric – is it scattered and inaccessible, centralized but with limited access, or centralized and accessible with robust governance? Also, consider how well you connect data insights to business goals. These factors paint a picture of your models' maturity
- And finally, to assess technology maturity, look at how your organization approaches data preparation and ML experimentation. You want to see if your organization has a central tool to give the right insight to the right person at the right time – or if you have a siloed approach wherein data scientists use local computers or servers that are difficult to obtain and set up
The answers to all these questions will lead you to your MLOps readiness score. With this data, you can create a visual representation to benchmark externally against competitors or internally across various business functions.
Assess maturity
When you understand all the areas of MLOps maturity, you can develop a questionnaire to evaluate your organization. All questions must carry a weighting that, when collated, will help you establish if your organization is of low, medium, or high maturity – in other words, a laggard, early adopter, or leader. Here's how:
- To assess people maturity, question how well you include the necessary data science and business leaders across all stages of the ML project lifecycle
- For process maturity, determine how you've organized your data fabric – is it scattered and inaccessible, centralized but with limited access, or centralized and accessible with robust governance? Also, consider how well you connect data insights to business goals. These factors paint a picture of your models' maturity
Understand maturity
Every organization is at a different stage when it comes to MLOps maturity. Therefore, it's essential to explore how your organization compares to industry averages and benchmarks to understand its potential for scalable ML solutions.
machine learning operations (MLOps)
Worse still, organizations spend precious time and resources monitoring and retraining models. Teams often can't replicate successful ML experiments, and data scientists don't have access to the technical infrastructure they need to innovate.